Blog
Humanizing data: How AI brings consumer persona to life
Explore how AI can help you create more engaging and accurate consumer personas.
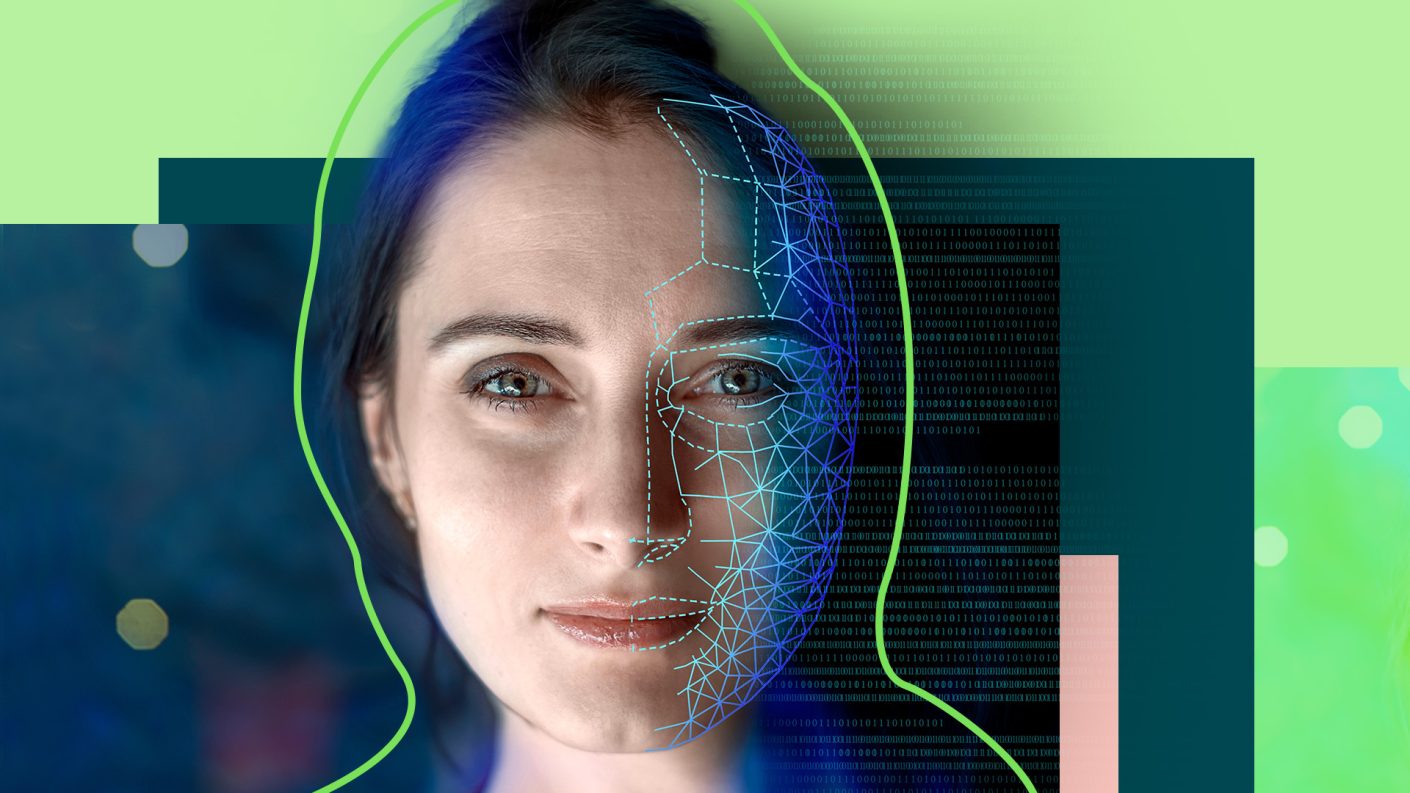
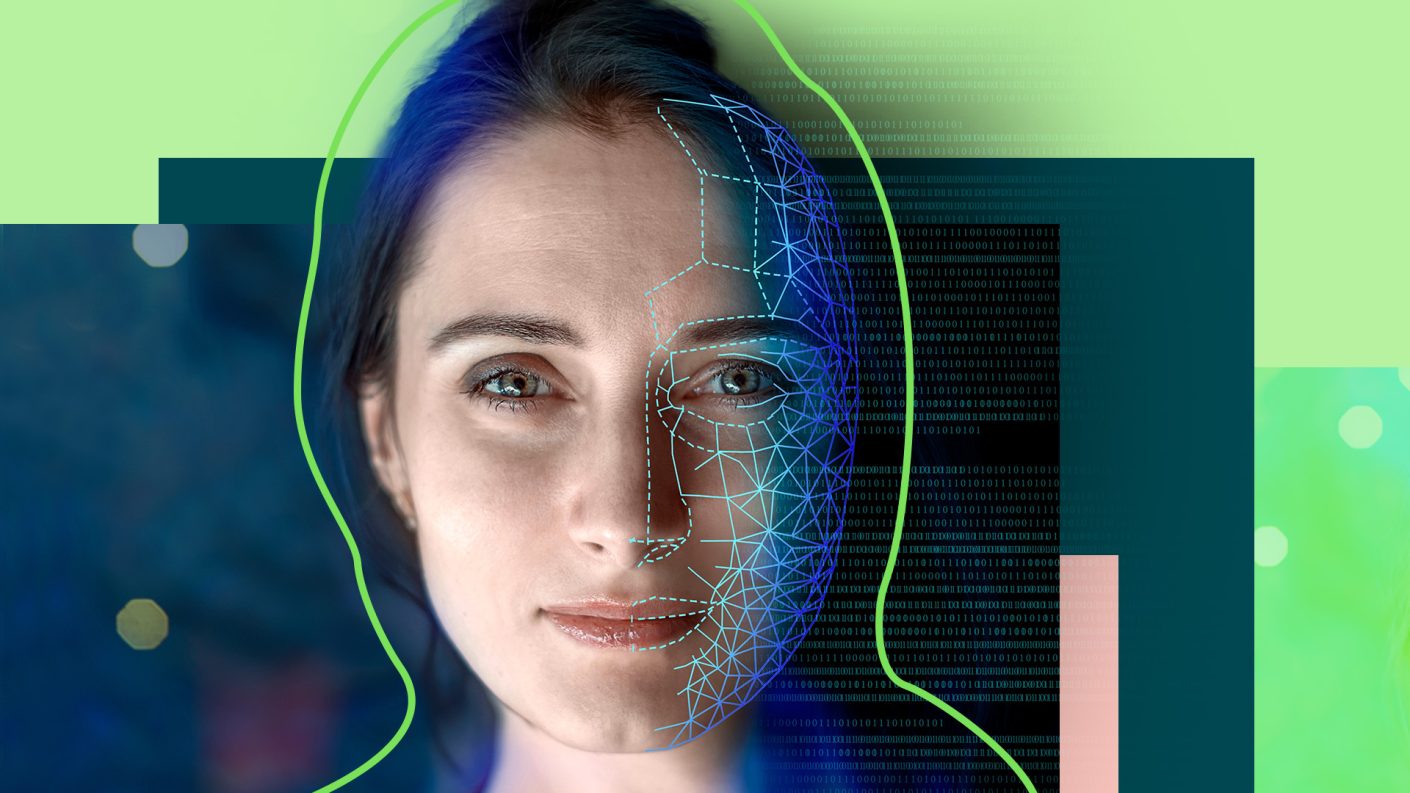
Annelies Verhaeghe
29 August 2024
6 min read
Imagine walking into a meeting room, eager to discover the results of your latest segmentation study. Instead of the usual presentation, you find yourself interacting with your brand’s personas through lifelike avatars. This might sound like a scene from the future, but with AI, it’s becoming a reality. AI is revolutionizing market research, offering innovative ways to create and engage with personas – those fictional characters that represent your potential customers. However, as we embrace this new technology, it’s important to understand the limitations of traditional personas and how AI can address these challenges.
The hidden flaws in traditional personas
Personas help you understand customers’ needs, experiences, behaviours and goals. But they also come with challenges. First, there’s a lack of details: a wealth of research and data gets distilled into an archetype, losing much of the detail and richness in the process, and complex concepts often become overly simplified. Although personas are based on primary research, those studies typically can’t cover everything we want to know about that profile. Imagine primary research probing deep into people’s car buying behaviour, but in hindsight you also would have loved to understand what kind of holiday type they are. This brings us to the issue of inadequate representation. We tend to fill in the blanks based on our own biases and perspectives, which can introduce stereotypes and assumptions. As such, different people might interpret the same persona profile in varied ways. Thinking back to our car example, reading the same persona you might think this is a type that goes camping, while I envision this more as a beach holiday type. This leads to inconsistencies in taking those personas forward. Lastly, there’s the issue of limited interaction: despite efforts to bring personas to life through methods like consumer museums, videos, or pictures, it remains hard for people to truly grasp and interact with the personas. It’s only when you engage with them that you truly understand the different profiles.
Detail and engagement: AI’s transformative impact on personas
This is where AI steps in, offering a transformative approach to persona development and activation. AI-generated consumer personas are digital representations of real people, designed to give brands a deeper, more nuanced understanding of their audiences. Unlike traditional personas, which often suffer from a lack of detail, AI personas are built using larger datasets. These capture a broader range of nuances by integrating all available research data, rather than just the key conclusions. This allows for a richer, more comprehensive view of the consumer. Moreover, AI brings personas to life in ways that were previously unimaginable, using chatbots, virtual avatars, and other interactive tools to make these profiles more engaging and memorable. This enhanced interactivity helps stakeholders truly immerse themselves in the consumer’s world, as noted by Bram Meijer, Marketing Director EMEA at Beyond Meat, who experienced this firsthand during a workshop with persona chatbots: “It was great to really immerse ourselves in the consumer’s world for a full day. It was a revelation for us how you can use AI for this. The chatbot was easy to use and provided rich insight, and the persona videos provided a nice summary to quickly understand our consumers.”
There are different ways to create such digital persona, either by relying solely on primary data, or by incorporating synthetic data. Your primary data might come from an online insight community where you tap into your consumers’ everyday lives, map out their customer journey or capture ethnographic data on how they use your product. This wealth of information can be used to create highly detailed consumer persona that internal stakeholders can consult via a chatbot, for instance. However, capturing every tiny detail of your target group’s lives is nearly impossible with primary research alone. As mentioned, there is a risk of filling in gaps with biases and perspectives, leading to stereotypes and assumptions. To address this, AI can bridge these gaps through synthetic data. While we primarily work with AI-generated personas based on primary data, we are also investigating the future potential of integrating synthetic data. In the remainder of this blogpost, we’ll explore how synthetic data could enhance the development of personas.
A glimpse into the future: Combining real and synthetic data for enhanced personas
Let’s start with highlighting the differences between real data versus synthetic data. In classic research with real data, we know that results are reliable because we have proven methodologies to handle bias. This is different for AI and synthetic data. In essence, synthetic data are statistical predictions based on training data. Imagine you want to understand how Alison – a 40-year-old school principal with a family of four who enjoys hiking – thinks about insurance. You prompt the generative AI system with your question and a detailed description of Alison. Based on the underlying Large Language Model (LLM), the system checks for people like Alison, what they say about insurance, and predicts what Alison might think. This prediction, or the synthetic data generated, will not be as good as real data, but it can complement it.
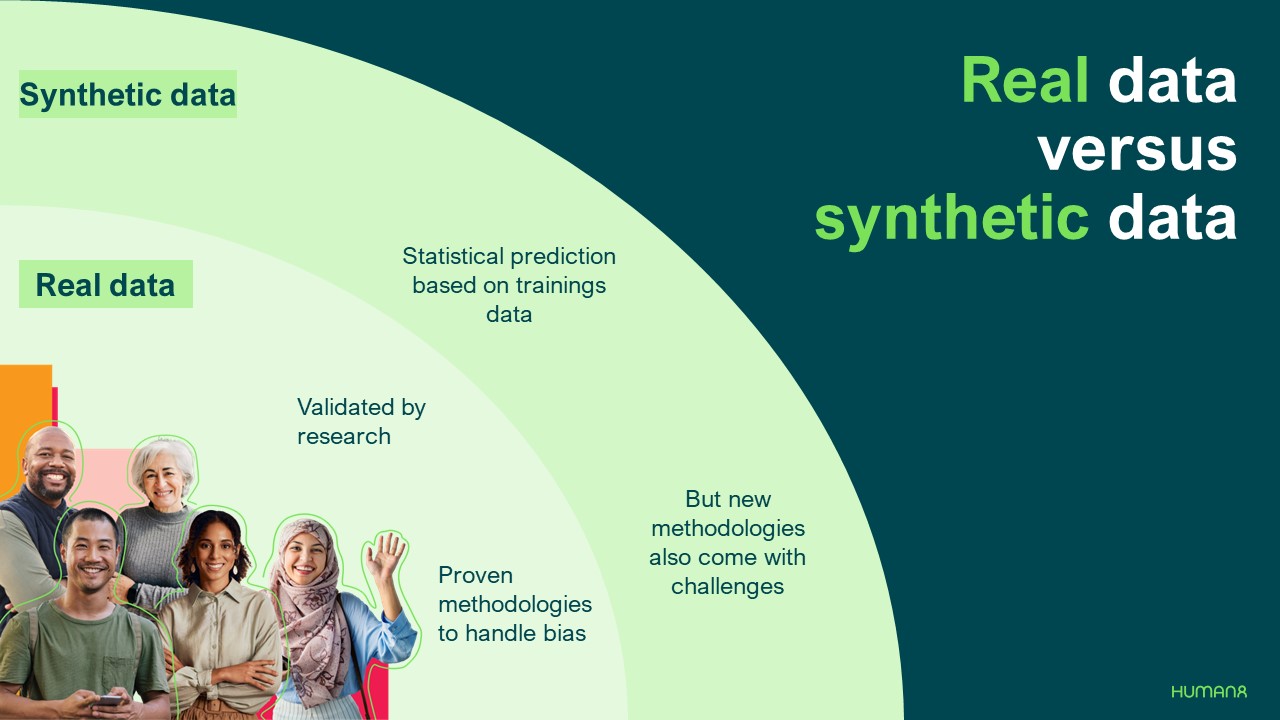
However, synthetic data also presents challenges. The first is that the result depends on the data used to train your model. The system is only as smart as the data it is trained on, which might result in biases, such as societal prejudices. For example, when asked for images of an “ambitious CEO”, the system might predominantly return pictures of males, while “supportive CEO” might yield more images of females.
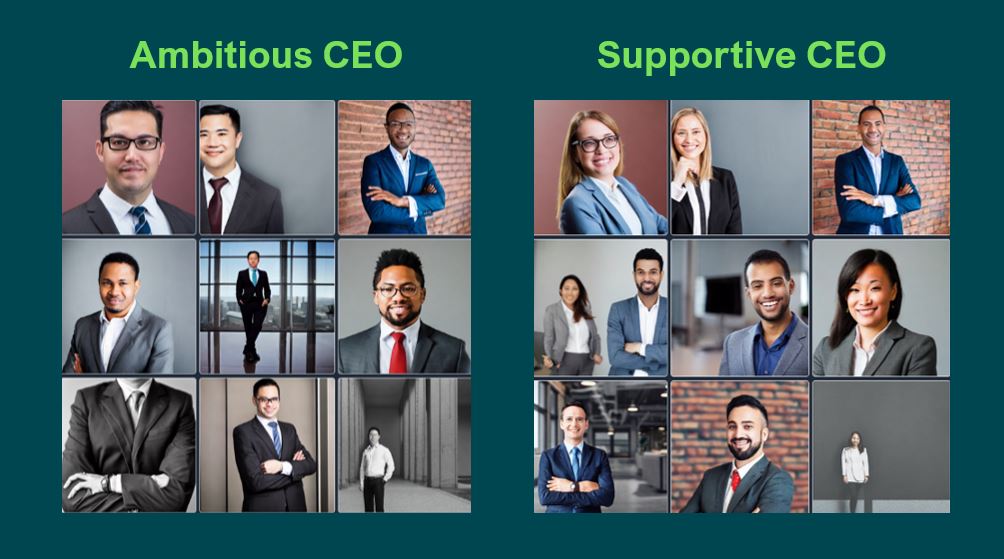
Second, the output largely depends on how you have prompted the system. Asking the right question is crucial to unlocking the full potential of generative AI, but crafting effective prompts is an art in itself.
To address these challenges, advanced methods of synthetic data creation are essential. We identify three key approaches: direct querying, data retraining and data augmentation.
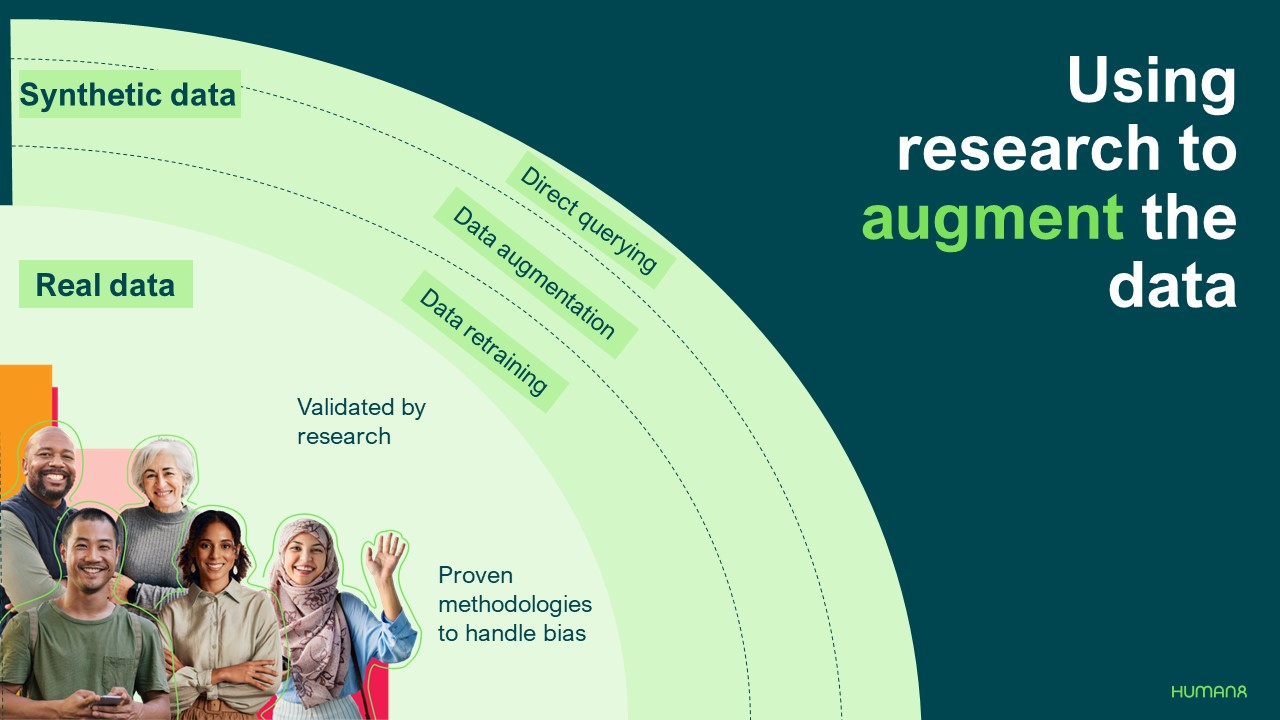
- Direct querying: This method generates pure synthetic data and is thus the furthest away from real data. Based on your own knowledge, you can describe a person within your target group and ask the AI system what that person would do, like, or think, very similar to our example of Alison. However, it’s important to approach this method with caution. In our initial experiments, we observed that the predictive power of pure synthetic data can be quite limited. For instance, when we conducted a correlation analysis comparing synthetic data-based prioritisations with ideas screened by actual consumers, the results showed a very low correlation, close to zero. While these findings are preliminary and should not be considered definitive, they do suggest that relying solely on synthetic data for predictions may not always yield accurate insights.
- Data retraining: This approach involves training models based on real, historical data which will improve its accuracy and predictive power. By tapping into extensive data archives, brands can better anticipate how consumers will react to new content or products. For example, some companies are now utilizing their historical data to predict consumer responses to advertisements or to generate visual heat maps that highlight where attention is focused in an ad. Similarly, many of our clients, including major retailers like Walmart, are increasingly sharing their data with suppliers. By leveraging this wealth of information, these brands ensure that their predictions are more closely aligned with actual consumer behaviours and market trends.
- Data augmentation: In the experimental work we do for our clients, we focus on data augmentation, which blends real and synthetic data. Rather than relying solely on synthetic data, we incorporate a layer of human data and understanding. For instance, in segmentation research, we create detailed personas based on our primary research before using querying. In our work with Beyond Meat, we used rich persona data to enhance the accuracy of synthetic data, bringing us closer to real-world insights. In our experiment around idea screening, we found very low correlations between synthetic data and real data. However, when we train synthetic data on personas from real-life online community participants, the correlation goes up to 0.52. While not perfect, starting with real data brings us significantly closer to reality. Careful persona crafting is thus essential in creating realistic synthetic data. Our experiments show that relying on deep qualitative data from real humans can significantly improve the predictive power of synthetic data.
To summarise, digital persona are powerful tools to put the consumer central within your company. These detailed, digital representations of real people help internal stakeholders to feel closer to consumers, better understand them and tailor strategies more effectively. While we primarily work with AI-generated personas based on primary data, we’re also exploring the future potential of integrating synthetic data. This glimpse into the future holds the promise of valuable insights that could complement traditional research, though it also presents unique challenges. The decision to incorporate synthetic data should be carefully weighed against the level of risk you’re willing to accept in business decisions. By thoughtfully blending synthetic data with real-world information, you can achieve more reliable and actionable insights. Ultimately, the key is to use synthetic data strategically, ensuring it enhances rather than replaces robust, primary research.
Want to explore digital persona?
Let’s connect