Blog
AI supercharges research
Four learnings on applying AI to supercharge data and insights.
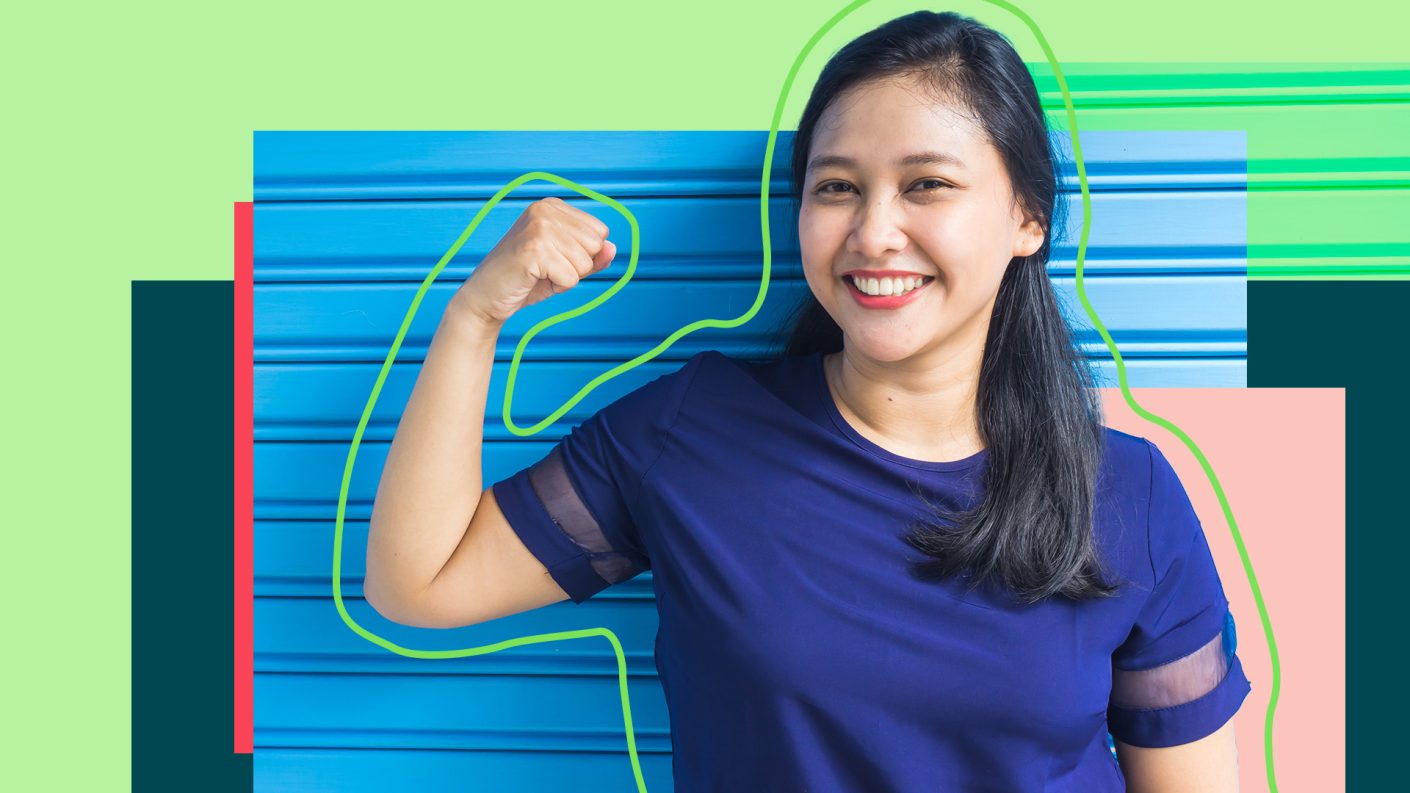
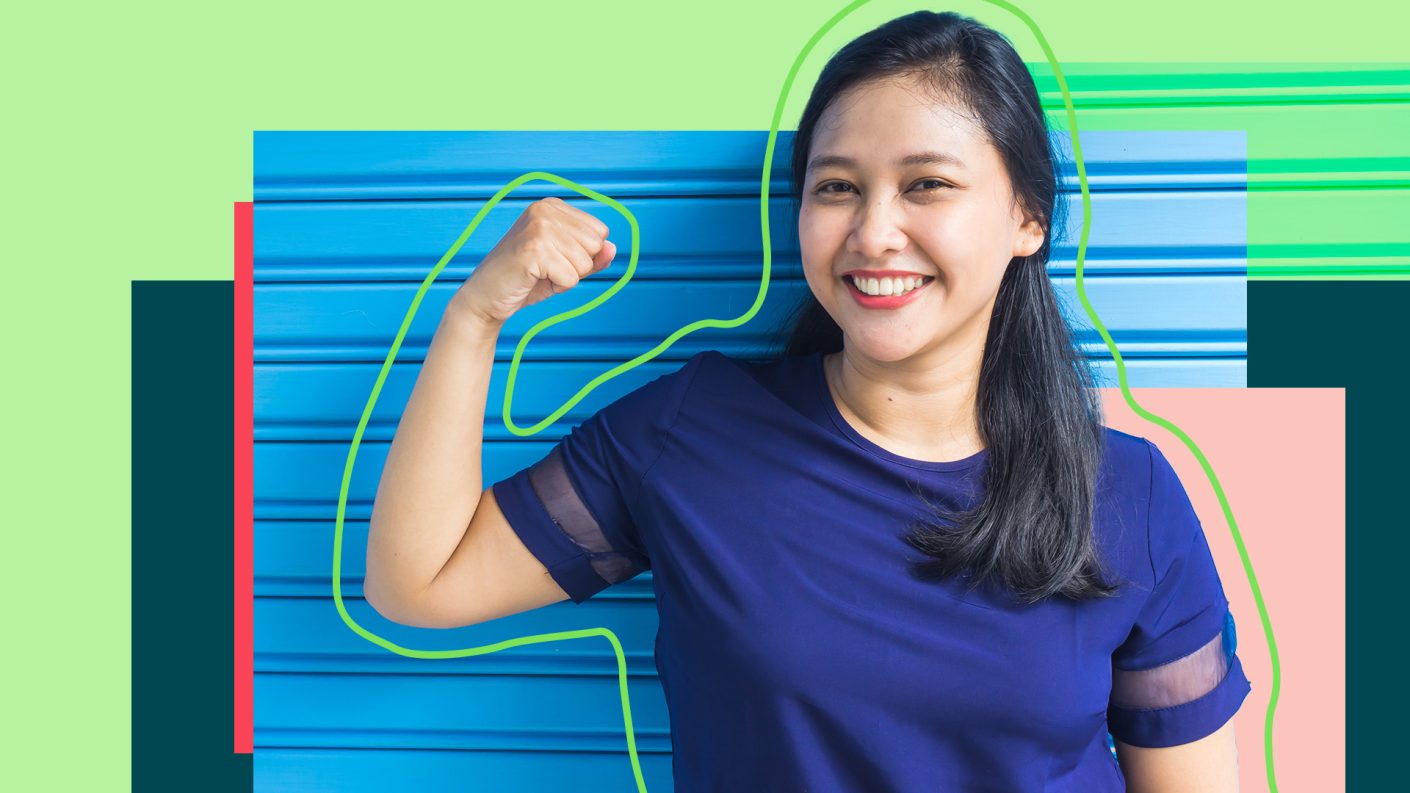
Annelies Verhaeghe
26 July 2023
4 min read
Since the introduction of ChatGPT in November 2022, generative AI is taking over the world (of insights) by storm. It’s the most talked-about topic among insight professionals with 93% of researchers seeing it as an opportunity for the industry. Esomar has set up an AI taskforce, MRS has written a white paper on AI regulation, and the AMA launched AI archives. But what does this mean for you as a brand or insight professional? Will primary research still be needed, or do we move to a world where AI systems will predict results without any research at all?
Exploring the future of research through AI experiments
We developed a personal research assistant – using ChatGPT as underlying algorithm – to safely experiment with generative AI in our day-to-day jobs. Here’s what we’ve learned.
1. AI lifts research to a higher level
From extracting insights, translating and summarizing research output in any language to creating surveys and topic guides, AI will help us become more effective in our everyday jobs.
At the start of a research project, these systems can also help us generate hypotheses, lifting the set-up and kick-off to a higher level. For example, we have used AI to predict the outcome of a concept validation piece on a new game. It retrieved a list of potential likes and dislikes that we took on board as hypotheses for this study.
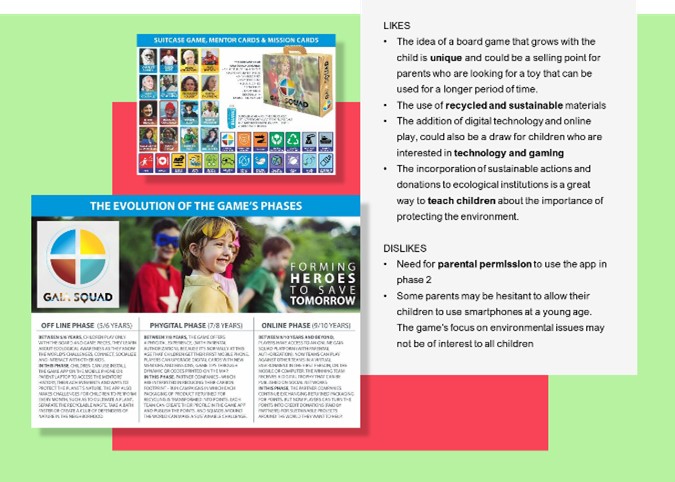
2. AI needs human eyes
To make AI work, it’s essential to feed the system with the right contextual information. Using AI effectively means we need to master the art of asking the right questions, fully understanding the client need, and prompting the system in the right way. We found that AI moderates responses in about 65% times brilliantly, but in 32% of the cases, it would give a prompt that was not relevant within the context of the research. When a respondent answered “no time” to the question “Why don’t you watch Netflix?”, the chatbot probed further with “What other forms of entertainment do you typically enjoy when you have free time?”. The question as such is not completely off, but not relevant in a study that focuses on the brand Netflix and not on entertainment in general.
3. AI needs human data
AI tools are very useful in the context of primary data gathering and analysis. We set up an A/B test where we asked our research assistant to use the knowledge of ChatGPT to find new insights on what well-being means for Gen Z in the greater Hong Kong Area. We also instructed the assistant to use its interpretative and analytics capabilities on community data that were gathered on this topic. We found that whereas the available knowledge of ChatGPT gave us a nice theoretical framework on well-being, the community data added an emotional, human layer that was far more actionable. The community data also revealed additional insights that were not picked up by our AI assistant.
4. AI helps to unlock the value of longitudinal data
Our experiments show that – when used in the right way – generative AI provides multiple opportunities to supercharge research. From project set-up to moderation and analysis, AI can play a significant role in each phase of a research project. But its true value doesn’t lie in the tool itself, but in the data it’s used on. Over time, AI systems can become real knowledge and insight assistants. They can easily curate and share insights across the many research projects you run each year. Just think about the data from ongoing research communities that have been running over multiple years. With one great prompt, you can get an answer to questions such as: ‘How has the perception towards sustainability changed for our three main target groups in five key markets?’. To be able to extract such meta-learnings AI tools need to be fed with high-quality data, meaning all underlying dimensions such as correct sampling, respondent quality, asking the right questions, … needs to be covered. It’s about feeding AI systems with high-quality (longitudinal) data to get to more relevant, interesting, and valuable output.
Machines need humans and humans need machines. AI tools supercharge human power, helping insight professionals do their jobs more efficiently. But we need to be careful in how we use them. If the basis is not covered – high quality (longitudinal) data and skilled human researchers – AI tools could potentially do more harm than good in guiding your business decisions. More experimentation is needed to fully grasp how to leverage AI as effectively as possible.
Let’s connect